Translation quality
Significantly better than generic models
Glossaries & Stop-Words as a Training Basis
Continuous Learning in Proofreading
High level of automation of training data acquisition
70% better translation result
Quality
70%
better
Translation quality
Significantly better than generic models
We tested:
How powerful is a custom-trained model compared to a generic model from DeepL?
Small spoiler – it’s 70% better translations.
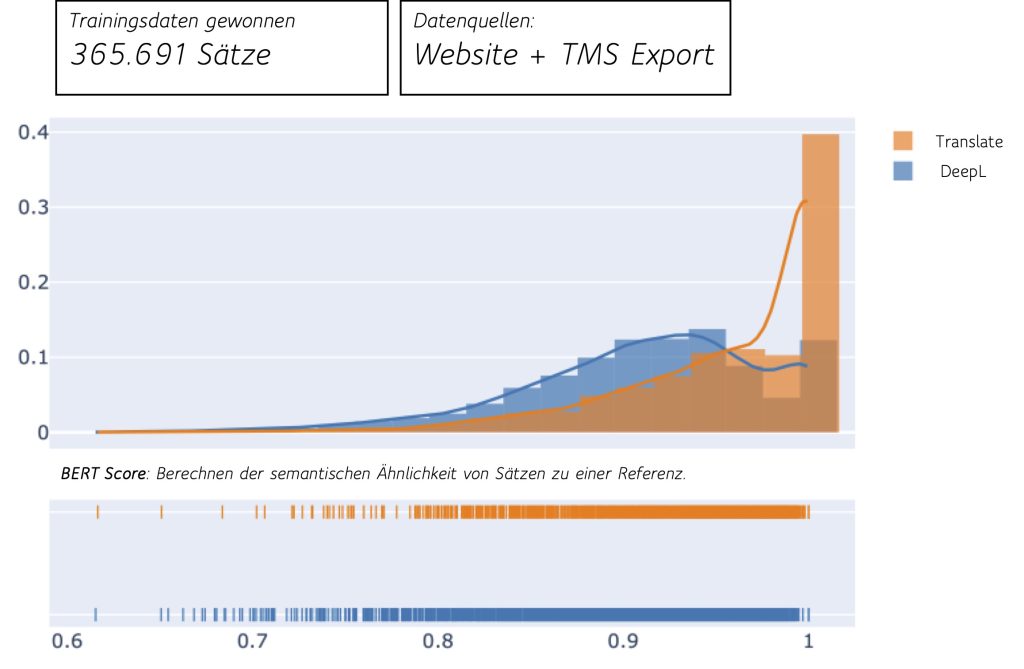
Test case (DE-EN):
The performance of the model trained by wonk.ai was tested against DeepL using the customer’s data.
In the customer area and in the customer-specific language, the model trained by wonk.ai was clearly convincing compared to DeepL.
2500 records were calculated from the customer domain.
365,691 sets won for training
Data Sources: Customer Websites & TMS Export
BERT Score for calculating semantic similarity to customer reference translation sentences
Better variant DeepL (sentences)
higher BERT score
Better variant DeepL (%)
higher BERT score
Better variant wonk.ai (sentences)
higher BERT score
Better variant wonk.ai
higher BERT score
Same Rating (Sentences)
Same BERT-Score
Same Rating (Sentences)
Same BERT-Score
We have mathematical models to assess a good translation.
But in the end, translations are communication,
between your business and the world – so let your team decide.
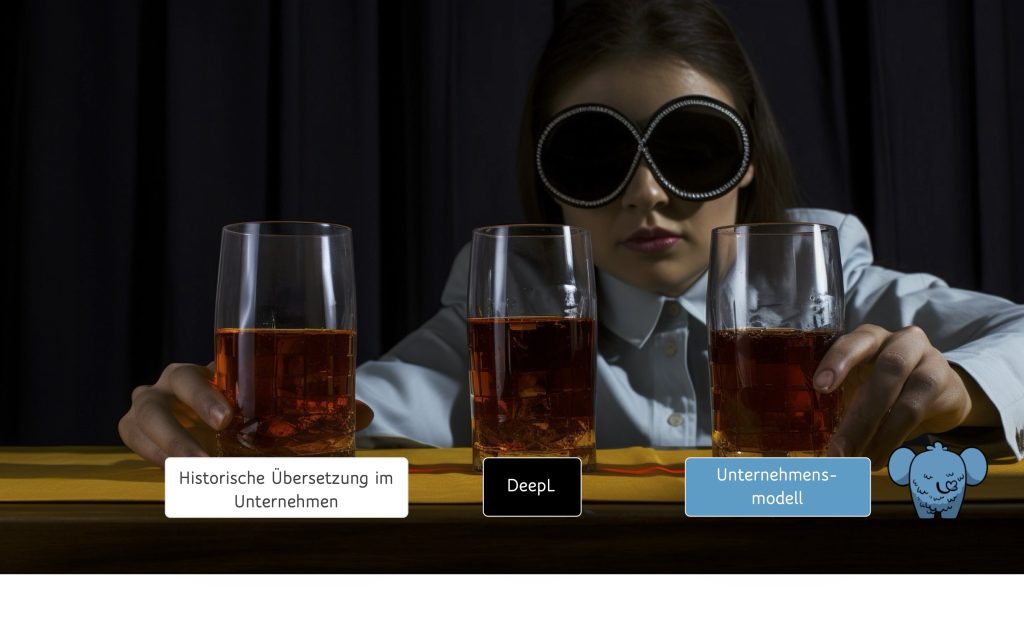
Decide on the quality.
Independent in your own evaluation environment.
After the model training, your stakeholders and translation managers will have their own access to your separate evaluation environment. There you can evaluate the quality of the translations without being influenced by the source.
In this way, you as the project manager receive the highest level of independent evaluation and, as a consequence, a very high level of acceptance of the trained models.
And if the evaluation is not positive?
This can happen – usually with not so strong basic models and little training data.
Then everyone involved knows right from the start that the models need even more quality.
With our continuous process of training data collection and enrichment, you can collect enough data over time to successfully train your own model.
Here’s how it works – Phase Plan Training & Operations
From data to translation
01
Data exchange
wonk.ai will receive the list of languages to be translated and access to the data sources available for training.
02
Data Checkup
wonk.ai checks the quality of the language data and the number of language pairs to be achieved and provides feedback on feasibility.
03
Specifying the testers
The client determines which stakeholders and experts in the company will review the language models and evaluate the results – compared to previous translations or alternative solutions.
04
Training of language models
wonk.ai extracts the language data, validates and cleans the training set, and trains the language models with mathematical evaluation.
05
Evaluation of the results
The customer’s testers evaluate the language models within their own assessment environment and provide feedback on individual results.
05
Commissioning
Once the language models have been initially approved, they can be put into operation directly and can be used via the customer’s own web environment. The trained models can also be integrated into third-party systems via the API and can thus be used in the entire system landscape.
Trained Translation Models
in company language. Mammothly strong.
Try Translate for free for 30 days!
Get your free access to real-time translations of texts and documents.
Your first step towards in-house translation models
Machine translation in the best possible quality.
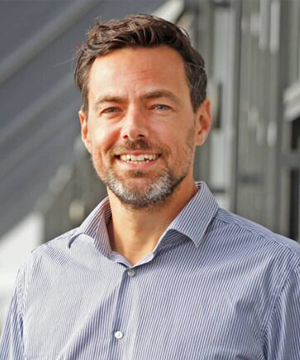
Any questions?
Time for a personal conversation.
We would like to help you and your company further and support you in the editorial department with AI. This often results in questions and topics that can be better clarified in the conversation. I’m happy to help you.
The quality fits…